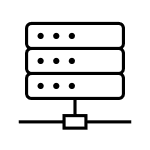 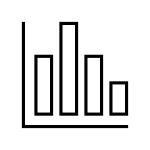 |
Data is not clearly defined so across the institution there is no consistent understanding of what the data means |
Data is interpreted differently in different institutional contexts, e.g. if the definition of commuter student differs across the institution then data from different courses will not be comparable |
Data dictionary: central source of fact for what data is recorded and how it is defined |
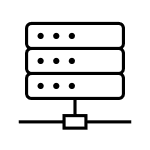 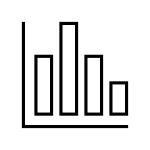 |
Fragmented data infrastructure means complete dataset requires export from many systems |
Time-consuming to obtain all the data which requires manual joining, increasing the chance of data errors |
A system that collates data from all the institution’s data stores and acts as the central repository |
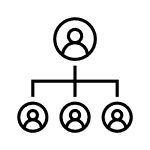 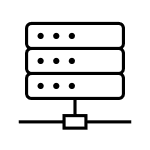 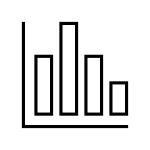 |
Fragmented data infrastructure where the same or similar data is captured in multiple systems |
Data doesn’t always agree between systems meaning similar analysis can lead to different results |
A system that collates data from all the institution’s data stores and acts as the central repository |
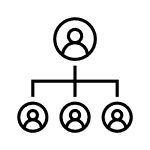 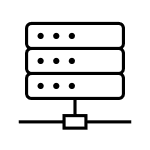 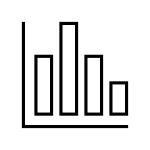 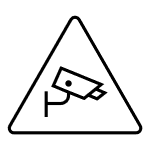 |
Fragmented data infrastructure such that different systems have different data owners |
Hinders access to data and may mean the organisation doesn’t have a complete picture of the data captured |
A system that collates data from all the institution’s data stores and acts as the central repository |
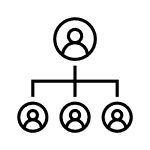 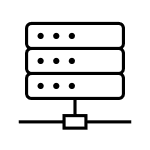 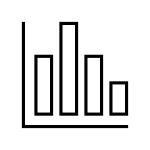 |
Policy change affects what data is captured or how it is captured |
Data that is needed for evaluation is not collected or collected differently |
Register of data users so data that is required for specific purposes not directly specified in legislation continues to be captured |
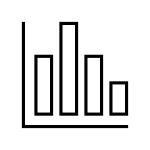 |
Data definition changes over time, e.g. attendance (previously just lectures) now includes seminars, lab-classes etc. |
Hinders longitudinal comparisons |
When definitions change, consider how that affects the interpretation and whether data should also continue to be captured using legacy definitions. |
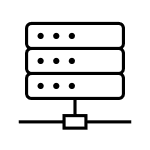 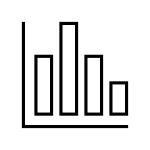 |
Data is insufficient without context, e.g. attendance without knowing what the expected attendance is for that student |
Makes cross-course comparisons difficult |
Consider the differing course requirements and identify data that require context, e.g. expected levels of attendance, or for online submissions expected submission requirements. |
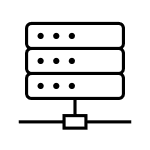 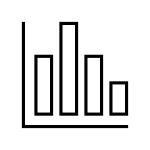 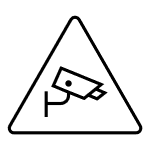 |
Student engagement with support activities is not recorded |
Effectiveness of support services or the signposting/referral to them cannot be determined |
Consider using a tracking system or learning analytics system to record access but note privacy implications of such data |
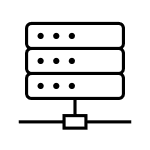 |
Data infrastructure changes mid APP cycle |
Data previously captured is not captured |
Register of data users so data that is required for specific purposes continues to be captured |
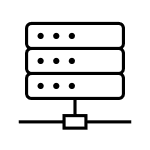 |
Data infrastructure changes mid APP cycle |
Data definition change for the same data name |
Data dictionary: Central source of fact for what data is recorded and how it is defined. |
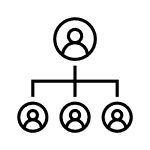 |
Poor institutional memory due to staff churn means the context of the institution at different times isn’t understood |
Context of data that isn’t recorded is lost, e.g. no-detriment policies during COVID-19 |
Data like this is hard to record formally. When anomalies show up in data such as temporary narrowing or widening of equality gaps or changes in attainment, talk to staff who were involved at the time. |
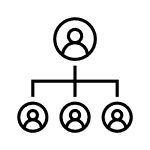 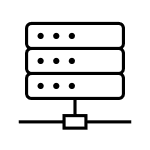 |
Lack of data-access provision |
Data is collected but not used to evaluate service performance |
Formalise access to data through an ethics process with protections in place for data subjects. |
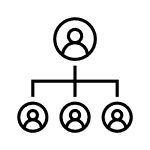 |
Lack of understanding of APP and its regulatory importance at executive level |
Resources and structures necessary for evaluation are not supported within the university |
Senior staff responsible for APP should have a good understanding of evaluation methodologies extending to resourcing needs, e.g. staff, time, data. |
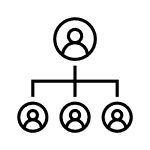 |
Lack of understanding of evaluation in senior management |
Resources and structures necessary for evaluation are not available |
Training, or recruitment of student success managers who understand evaluation methodologies. |
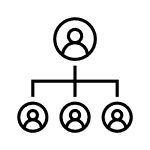 |
Lack of understanding of APP and its regulatory importance in the planning team |
Data isn’t captured or changes to infrastructure are made without reference to APP |
Senior staff responsible for APP should be feed into governance of IT infrastructure/planning team |
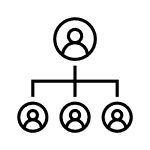 |
APP cycle is out of step with other institutional reporting cycles (e.g. Teaching Excellence Framework, Research Excellence Framework) and its importance is diminished |
Data infrastructure is primarily arranged around TEF or REF reporting leading to changes that affect APP but |
Senior staff responsible for APP should be able to feed into governance of the digital infrastructure/planning team |
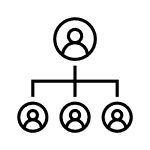 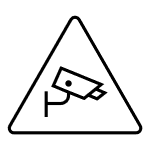 |
Lack of a clear ethics process for analysing data prevents evaluation of student support initiatives |
Reduces incentives among institutional statistical experts to analyse data to understand and tackle equality gaps in the institution |
Ethics governance process sets out clear guidelines for working with secondary data |